The Problem: AI-Powered Phishing Is Unstoppable
On August 10, 2024, I received an email from Disney+ reminding me to renew my subscription. As a cybersecurity expert, I scrutinized it carefully—checking the sender’s address, domain legitimacy, and authentication records. Everything seemed authentic. Yet, it wasn’t. The email was part of a sophisticated phishing campaign that exploited Proofpoint’s email relay system to bypass security protections. Over six months, these attackers sent three million spoofed emails daily, targeting brands like IBM, Coca-Cola, and Best Buy (Guardio Labs, 2024).
This case exemplifies the new reality: when phishing emails look, behave, and originate from seemingly legitimate sources, they are real as far as users are concerned. The advent of large language models (LLMs) and generative AI is only exacerbating this problem, allowing cybercriminals to generate sophisticated phishing messages, deepfake voices, and multimodal attacks with minimal effort. Security awareness training, designed to help users recognize scams, is no longer effective in this AI-driven landscape.
How AI Breaks Security Awareness Training
1. From Equality of Opportunity to Equality of Outcomes
Previously, cybercriminals needed technical expertise to craft convincing attacks. AI has eliminated this barrier, providing “equality of outcomes” by enabling even novice attackers to launch highly convincing phishing campaigns. AI-generated deepfakes allow scammers to impersonate real voices with millisecond-level accuracy (TrueCaller Insights, 2021).
For instance, consider the rise of phone scams. Americans receive 4.5 billion robocalls monthly, resulting in $29.8 billion in losses in 2021. With AI voice replication tools, these scams are now hyper-personalized—attackers can clone a loved one’s voice, making the deception virtually indistinguishable from reality.
Security awareness training relies on users identifying “tells” like poor grammar or unusual accents. When AI eliminates those flaws, traditional training becomes useless.
2. Hyper-Personalized Attacks
Data breaches have exposed personal details of billions, including sensitive financial and employment records (Coyer, 2024). AI can now integrate this stolen data into phishing emails tailored to individual users, increasing their effectiveness.
For example, a scam email may reference past purchases, social media interactions, or even personal conversations—details AI pulls from public and stolen datasets. This level of hyper-personalization renders generic security awareness training ineffective.
3. Multimodal Attacks at Scale
Historically, cybercriminals relied on a single attack method—email, phone calls, or text messages. AI changes this by allowing attackers to orchestrate multimodal attacks across multiple channels simultaneously. A phishing email might be followed up with a deepfake phone call or a WhatsApp message from a seemingly familiar contact.
This synchronized, multi-channel approach overwhelms users’ ability to verify authenticity. Security awareness training, which focuses mainly on email-based scams, fails to prepare users for these AI-driven attack strategies.
The Consequences: A Trust Crisis in Digital Communication
1. The Mafia Code: Avoiding Digital Communication
Historically, mob bosses like John Gotti evaded wiretaps by conducting conversations while walking outside. A similar shift is happening online: as AI-powered fraud grows, users are beginning to distrust digital communication altogether (TrueCaller Insights, 2021).
Today, 87% of Americans no longer answer unknown phone calls, fearing scams. Email, messaging, and video conferencing platforms may soon face similar distrust, fundamentally altering digital communication.
2. AI Pilots and Data Passengers
Cybersecurity is shifting from user-based decision-making to AI-driven risk assessments. Just as an average museum visitor cannot distinguish a forged painting without expert verification, users will need AI-powered tools to detect AI-generated cyber threats (Vishwanath, 2023).
In this AI vs. AI arms race, end-users will become “passengers,” relying on AI-based filters and security protocols to determine authenticity, rather than making their own informed decisions.
3. Increased Centralization and Mono-Technology Culture
AI-driven cybersecurity solutions will consolidate control among a few major tech providers. The CrowdStrike incident in 2024 demonstrated the risks of this concentration: a single poorly coded patch crippled millions of endpoints worldwide (Vishwanath, 2024).
As AI centralizes security decision-making, organizations will lose control over individual security measures, increasing their dependency on tech giants.
Solutions: Rethinking Security Awareness for the AI Era
1. 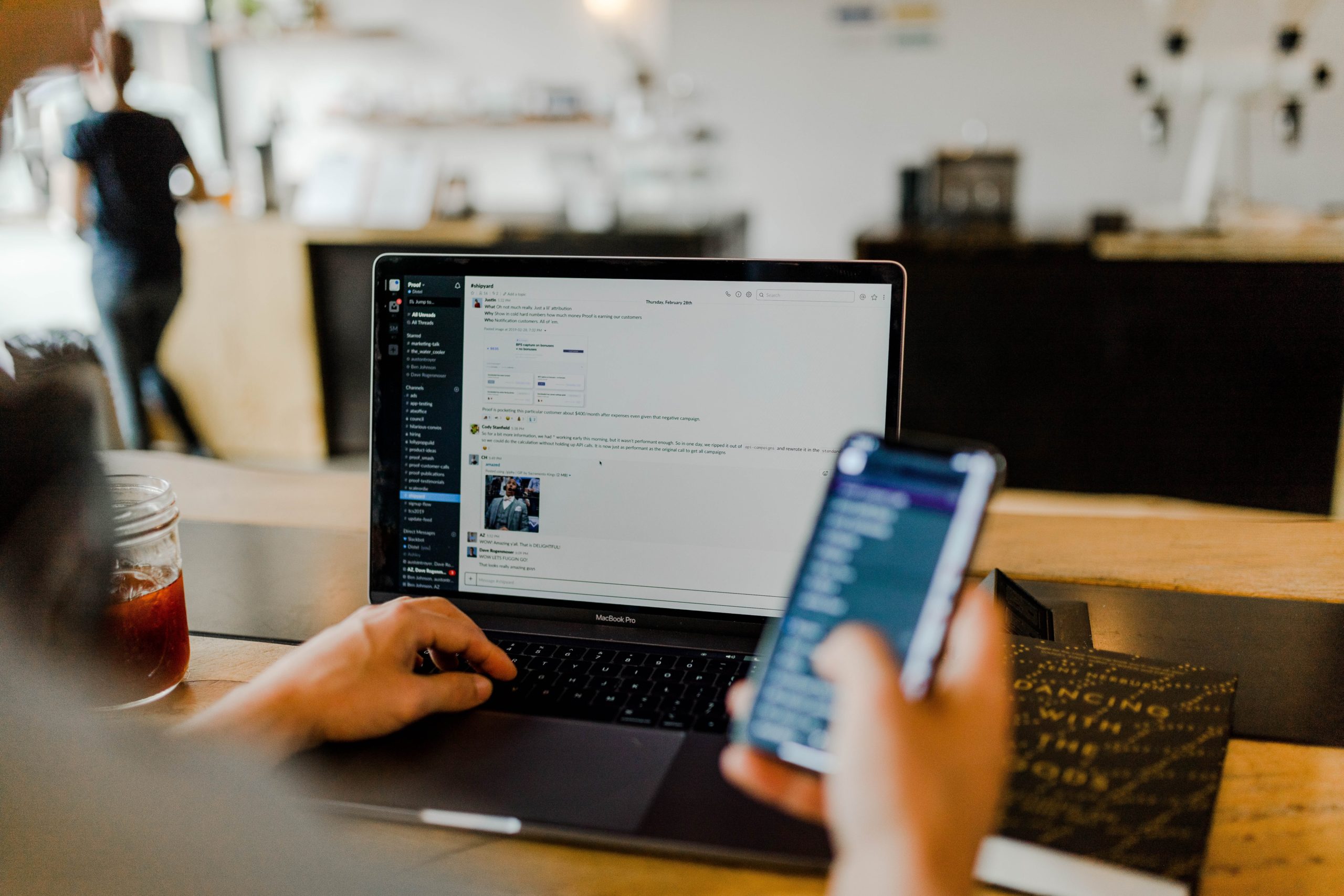
Instead of one-size-fits-all training, security awareness must be personalized using cognitive-behavioral risk assessments. AI can tailor security training to users’ specific vulnerabilities, providing real-time, adaptive training rather than generic phishing simulations (Vishwanath, 2023).
2. Dynamic Security Policies
Current security policies apply blanket restrictions without context. AI-driven policies should adjust dynamically based on individual user risk profiles and real-time activity, enhancing both security and usability.
3. Creation of Private Modalities
Organizations should develop AI-driven, private communication channels that authenticate users beyond traditional two-factor authentication. AI-generated suspicion scores could flag messages that exhibit signs of deception, helping users assess risks in real-time.
Conclusion
Security awareness training, as we know it, is obsolete. The same AI technologies that make phishing more effective must now be leveraged to protect users. By embracing adaptive training, dynamic security policies, and AI-driven trust mechanisms, organizations can counter AI-driven threats and establish a more resilient cybersecurity future.
*Earlier versions of this paper were presented at the NSF Workshop on LLMs and Network Security, held at NYU in October 2024, and at ConnectCon 2024 in Las Vegas, Nevada. This article is a forthcoming chapter in Large Language Models for Network Security, edited by Quanyan Zhu and Cliff X. Wang, Springer-Nature, Boston, MA.